Talk: Privacy-Preserving Data Sharing in Intrusion Detection Systems, 12/6 online
12–1pm EST Friday, December 6, 2024, online
UMBC Cyber Defense Lab presents
Privacy-Preserving Data Sharing in Intrusion Detection Systems
Zhiyuan Chen
Professor and Chair, UMBC Information Systems Department
12:00–1pm, Friday, December 6, 2024, online
Intrusion detection systems increasingly use machine learning methods, which require large volumes of data to be effective. Sharing such data sets will benefit the research community and industry. One obstacle to sharing such data is data privacy because network trace data or server log data often contains sensitive information, such as IP addresses. Even if IP addresses are encrypted, adversaries may still inject packets with unique patterns (e.g., with a certain packet sizes) such that they can use these packets to infer encrypted information. Another challenge arises when multiple intrusion detection systems from multiple organizations need to correlate their detected alerts to identify a larger threat, but the information they exchange may contain sensitive information such as network topology and traffic. This talk covers two approaches to address this problem. First, we propose a data anonymization approach that de-identifies network trace data. Compared to existing approaches, this approach provides stronger privacy protection and is robust to injection attacks. Second, we propose two privacy-preserving distributed alert correlation methods, one using additive secret sharing and the other using differential privacy. We also investigate tradeoffs between these two methods.
Dr. Zhiyuan Chen is a Professor in the Department of Information Systems at UMBC. He received a BS and a MS from Fudan University, China, and a PhD in Computer Science from Cornell University. His research covers the areas of data science, big data, privacy preserving data mining and data management, data exploration and navigation, and semantic-based search and data integration using semantic networks, adversarial learning and its applications in cybersecurity. He has published extensively in these areas and has received funding from NSF, Department of Energy, IBM, Office of Naval Research, MITRE, and Department of Education.
Host: Alan T. Sherman. Support for this event was provided in part by NSF under SFS grant DGE-1753681. The UMBC Cyber Defense Lab meets biweekly Fridays 12-1pm. All meetings are open to the public.
Posted: November 29, 2024, 5:28 PM
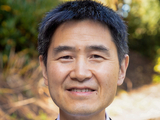